How is generative AI revolutionizing content creation and innovation? This guide cuts through the complexity, offering you a clear understanding of how these AI systems work and their real-world applications. Explore the present impact and future potential of generative AI across industries without getting lost in technicalities.
Table of Contents
Key Takeaways
Generative AI leverages neural networks and machine learning to generate diverse content including text, images, audio, and synthetic data, employing complex algorithms to create unique, realistic content.
The applications of generative AI are vast, revolutionizing industries by enhancing content generation, automating business processes, and transforming companies’ operational strategies.
Ethical considerations are paramount in generative AI development, focusing on managing risks associated with AI-generated content, ensuring fairness, and reducing bias to foster responsible AI adoption.
Exploring the Fundamentals of Generative AI
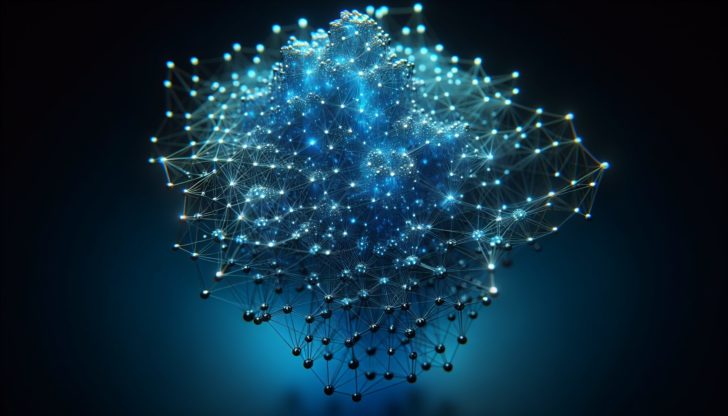
Generative AI is anchored in the principles of neural networks and machine learning models. Through these, it can generate a wide range of content, including:
Text
Images
Audio
Synthetic data
One such example is the generative AI model, which utilizes machine learning and neural networks to create diverse outputs.
This is achieved by recognizing patterns and structures within existing data and employing complex algorithms to create fresh, unique content.
The Role of Neural Networks in Generative AI
Generative AI is built on the foundation of neural networks. These networks abstract intricate, high-level data patterns, a crucial factor in producing lifelike content. By recognizing and understanding data structures, they aid in the creation of novel content through a variety of generative AI models.
Machine Learning Models Powering Generative AI
Generative AI utilizes a range of machine learning models, including other generative AI models, each with unique methodologies and operational principles. These include GANs and VAEs, which prioritize creating data that closely resembles real data and producing new samples from a learned latent space, respectively.
The Mechanics Behind Generative AI Systems
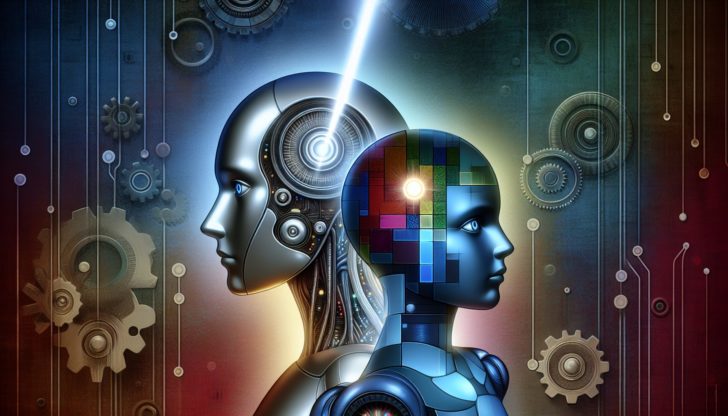
Generative AI mechanics encompass learning from data to spawn creative content. Foundation models such as GPT-3 and DALL-E significantly contribute to this field’s progression. Training models to discern patterns and structures in the data is a part of the learning process, which uses these patterns as a springboard for content creation.
From Data to Creativity: How Generative AI Learns
The learning procedure in generative AI leverages neural networks to discern patterns and structures within training data. The AI learns to create authentic samples via methods such as adversarial training, consequently leading to the generation of creative content.
The Evolution of Foundation Models in AI
Models like GPT-3, known as foundation models in AI, offer a flexible base for developing more specialized models, including generative models. As one of the most prominent large language models, they provide robust frameworks that can be tailored for diverse applications by training on extensive datasets, thus propelling the growth of generative AI.
The Spectrum of Generative AI Applications
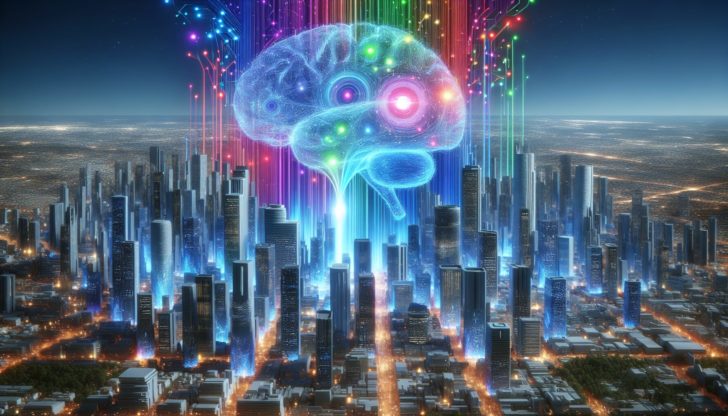
The applications of generative AI span a wide range of industries, revolutionizing content generation and business processes. From logistics and transportation to healthcare and education, generative AI is transforming the way companies operate and deliver value.
Revolutionizing Content Generation with AI
Generative AI is revolutionizing content generation in a multitude of industries, including image generation. It fosters the creation of engaging content in real-time in domains like journalism, literature, and music, paving the way for tailoring tracks to suit a diverse audience.
Innovative Uses of Generative AI in Business Processes
Innovative applications of Generative AI are visible in numerous business processes. It is elevating efficiency and productivity across sectors by automating customer support, content marketing, and generating realistic training media.
Implementing Generative AI Solutions for Competitive Advantage
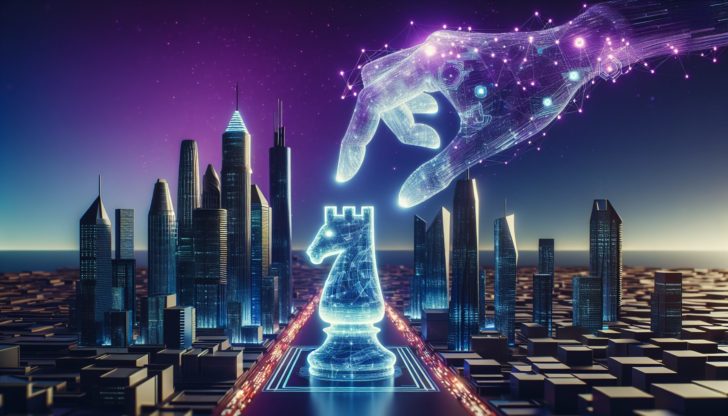
Businesses can gain a competitive edge by implementing generative AI solutions. They enhance process automation, workflow optimization, and personalized content creation, resulting in cost reduction and better customer engagement.
Customizing Generative AI for Unique Business Needs
Customizing generative AI for specific business needs can enhance its effectiveness. By identifying specific areas where generative AI can add value, collecting relevant data, and developing tailored models, businesses can better leverage the benefits of generative AI.
Integration Strategies for Generative AI in Existing Workflows
The potential of generative AI is maximized when integrated into existing workflows. Conducting comprehensive client research, breaking down tasks into unique components, and tailoring the final outputs allow businesses to smoothly incorporate generative AI models into their workflow processes.
The Ethical Implications of Generative AI
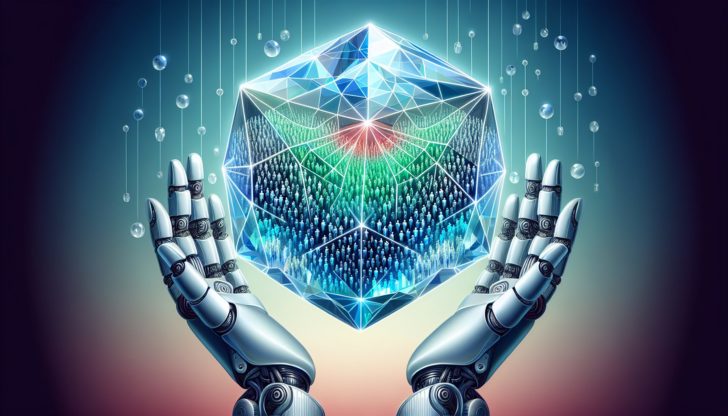
Ethical implications of generative AI encompass risks linked with AI-generated content and the necessity for fairness and bias reduction. Addressing these ethical considerations is of utmost importance for responsible AI adoption as generative AI continues to evolve.
Navigating the Risks of AI-Generated Content
It’s critical to manage the risks linked with AI-generated content. Adherence to ethical guidelines, emphasis on social benefit, avoidance of unfair bias, safety assurance, and maintaining accountability can ensure organizations responsibly use AI-generated content.
Ensuring Fairness and Reducing Bias in AI Systems
Ensuring fairness and reducing bias in AI systems is essential for ethical AI implementation. By using metrics that assess the model’s fairness and implementing techniques to address bias, organizations can create more equitable AI systems.
Advancements and Future Directions in Generative AI Research
The future of technology is being molded by advancements in generative AI research, including natural language processing. The recent progression in language models and the emergence of small language models position generative AI to redefine the horizons of creativity and innovation.
Breakthroughs in Generative AI Techniques
Breakthroughs in generative AI techniques, such as advanced image generators and emotionally intelligent chatbots, are driving innovation and opening up new applications across various fields. These advancements are not only enhancing the capabilities of generative AI, but they are also enabling the development of novel solutions for complex problems.
Visionary Predictions: The Next Frontier of Generative AI
Visionary predictions suggest a transformative future for generative AI. As the technology continues to evolve, it is anticipated to have a significant influence on various industries, with potential technological advancements reaching human-level performance.
Learn more, visit The future of generative AI? An early view in 15 charts.
Maximizing the Benefits of Generative AI
Maximizing the benefits of generative AI involves not only leveraging the technology to enhance creative capabilities but also using it to improve decision-making and problem-solving processes.
By understanding the full potential of generative AI, businesses can optimize its benefits and create value.
Enhancing Creative Capabilities with Generative AI Tools
Generative AI tools empower creativity and innovation in various fields. These tools include:
DALL-E 2
Scribe
Jasper
ChatGPT
Replit
They enable collaborations between designers and users, assist in the co-creation of new offerings, and serve as an inexhaustible source of inspiration for generating a diverse range of innovative ideas through stable diffusion.
Decision-Making and Problem-Solving with Generative AI
Generative artificial intelligence has the potential to:
Dramatically improve decision-making and problem-solving processes
Organize and classify data
Offer significant insights
Generate artificial data
By utilizing generative AI, you can find solutions to complex problems and make more informed decisions.
Generative AI in Action: Real-World Success Stories
The impact of generative AI adoption across diverse industries is demonstrated through real-world success stories. They underscore the transformative role generative AI plays in the modus operandi of companies in fields including:
Logistics
Healthcare
Education
Banking
These success stories highlight how generative AI enhances value delivery.
Trailblazers in Generative AI Adoption
Trailblazers in generative AI adoption, such as:
Microsoft
FedEx
Marriott Hotels
Metro Bank
Expedia
are reaping the benefits of this technology. Through successful implementation, these industry leaders are demonstrating the potential of generative AI to transform business operations and deliver value.
Measurable Outcomes from Using Generative AI
Measurable outcomes from using generative AI demonstrate its value and potential. From enhancing labor productivity to improving the performance of highly skilled workers and optimizing business workflows, generative AI is proving to be a valuable asset in achieving business objectives.
Summary
In conclusion, generative AI is a revolutionary technology that is transforming industries and redefining the boundaries of creativity. From enhancing creative capabilities and improving decision-making to facilitating ethical AI adoption and driving innovation, the potential of generative AI is vast. As we continue to explore this technology, it is crucial to navigate its risks, maximize its benefits, and harness its full potential.
Frequently Asked Questions
What is generative AI vs normal AI?
Generative AI creates new data based on what it’s learned, like images, music, or stories, while traditional AI analyzes existing data to find patterns and make predictions. Traditional AI is like a data wizard, while generative AI crafts new content.
What is the most famous generative AI?
The most famous generative AI models include ChatGPT and OpenAI’s DALL-E 2, which are widely recognized for their text and image generation capabilities.
How does generative AI work?
Generative AI works by using neural networks to analyze existing data and creating new content based on recognized patterns and structures. This process involves complex algorithms to generate unique outputs.
How can generative AI benefit businesses?
Generative AI can benefit businesses by automating processes, optimizing workflows, and creating personalized content, ultimately leading to reduced costs and improved customer engagement. Embracing this technology can significantly enhance business operations.
Are there any ethical concerns associated with generative AI?
Yes, there are ethical concerns associated with generative AI, such as risks related to AI-generated content and the importance of fairness and bias reduction.